Artificial intelligence: Revolutionising protein structure prediction

Rohini Karandikar
Consultant,
TNQ Foundation
02-April-2025
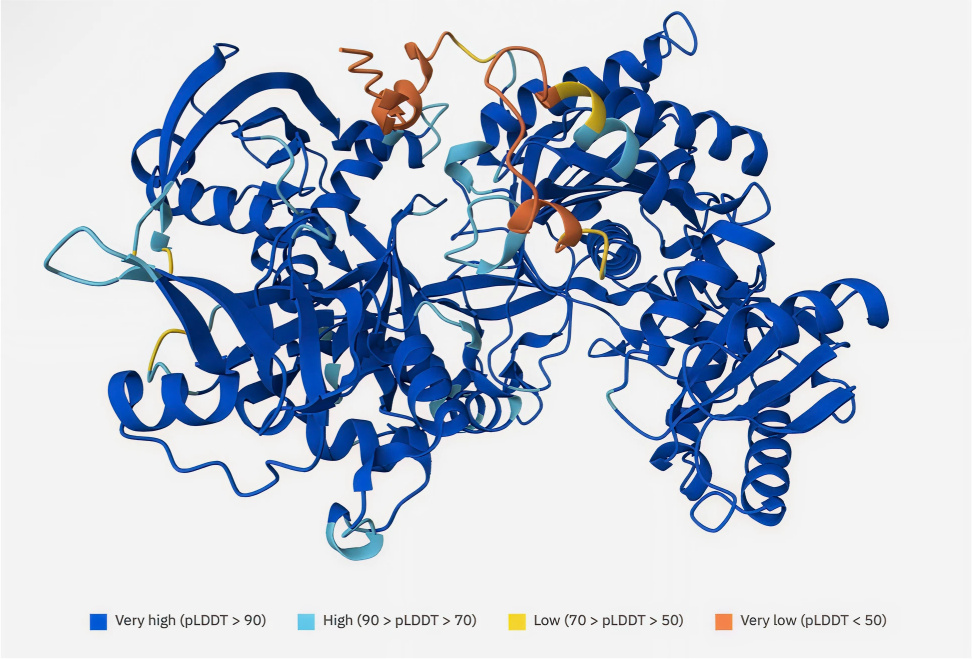
Life on Earth would not have existed without proteins. Proteins are
made up of amino acids that join to form polypeptide chains. The
chains fold in a specific manner that depends on the sequence,
location of all amino acids, and their interactions. All these
factors determine the three-dimensional structure of a protein,
critical for the protein's function. Whether it is the spike
glycoprotein of the SARS-CoV-2 or the hemoglobin that carries oxygen
in our blood, it is functional due to its structure.
Why is knowing a protein structure important?
Scientists are interested in determining the three-dimensional
structures of proteins, as the structure gives insights into how
proteins interact with other proteins and macromolecules. The
structures also help understand the mechanisms of diseases and
enable the effective design of drugs and diagnostics. Knowledge of
protein structure helps in crucial applications such as developing
proteins and enzymes for industrial applications and vaccines
against pathogens.
How did protein structure prediction evolve over the
years?
In the mid mid-1950s, Christian Anfinsen was the first to establish
a link between a protein's amino acid sequence and its
three-dimensional structure, through his work on the enzyme
ribonuclease. He proposed a hypothesis which states that a protein's
structure can be predicted from its amino acid sequence and the
environmental conditions in which the protein folds.
A few years later, John Kendrew and his team elucidated the first 3D
structure of a protein— myoglobin, the oxygen-storing protein in
muscle cells, in 1958 using X-ray crystallography. Kendrew and Max
Perutz jointly received the Nobel Prize in Chemistry in 1962 "for
their studies of the structures of globular proteins." Later, in
1972, Anfinsen won the Nobel Prize in Chemistry for his work on
ribonuclease.
For a long time, researchers would experimentally determine protein
structures using other techniques, such as cryo-electron microscopy
and nuclear magnetic resonance (NMR) spectroscopy. However, these
techniques required expensive equipment and posed computational
challenges in predicting 'lowest free energy conformations,' as
proteins can potentially fold into a vast number of conformations.
Importantly, these methods required days, months or even years to
predict proteins structures. Further, some proteins, such as
membrane proteins, are difficult to crystallize, and their structure
determination is difficult. As a result, out of ~23 crore protein
sequences available, the structures of only about 2,00,000 proteins
were known.
Enter AlphaFold. In 2020, Demis Hassabis, an artificial intelligence
researcher and John Jumper, a chemist and computer scientist
presented an artificial intelligence-based solution to the problem
of predicting three-dimensional structures of proteins. Their
model—AlphaFold2
by
Google DeepMind,
was awarded the 2024 Nobel Prize in Chemistry, which they shared
with David Baker for his work on computational protein design.
Earlier in 2024, John Jumper delivered a TNQ Distinguished Lecture
on “Highly accurate protein structure predictions: Using AI to solve
biology problems in minutes instead of years” in Bengaluru and
Mumbai, India.
How does AlphaFold2 work?
AlphaFold2 is a tool that uses artificial intelligence and requires
the amino acid sequence of a protein as input in the first step of
protein structure prediction. AlphaFold2 is trained in recognizing
patterns from known protein structures from databases like
UniProt
or the
Protein Data Bank
(PDB). The PDB is a database consisting of three-dimensional
structures of biological macromolecules, including those of
experimentally determined protein structures. In the next step,
AlphaFold2 explores which amino acids are likely to interact with
each other in the 3D structure. The AI model then uses neural
networks to map the relative distances of amino acids. Finally, in
an iterative process, AlphaFold2 generates an accurate
three-dimensional protein structure.
AlphaFold1—the earlier version and AlphaFold2 both competed in what
is known as the "Olympics of Protein Folding"-- the
Critical Assessment of Structure Prediction
(CASP), a global competition, where researchers predict
3D-structures of proteins purely based on the proteins' amino acid
sequences. The participants are given amino acid sequences of
proteins whose structures have just been determined experimentally,
but these structures are kept secret. AlphaFold2 aced the
competition in the 14th edition of CASP in 2020, accurately
predicting protein structures and outperforming other methods.
The future with AlphaFold2
Today, AlphaFold2 is available in the open domain; its source code
is freely available online to expedite research in the life
sciences. During the COVID-19 pandemic, AlphaFold2 accurately
predicted the structure of proteins of SARS-CoV-2 and enabled
sharing those with researchers globally. This promoted faster
vaccine design and accelerated drug development.
AlphaFold2 has nearly solved the major challenges of protein
structure prediction, drastically reducing the cost and time
required for structure prediction using experimental methods. The
researchers of AlphaFold have created a database of more than 200
million protein structures, covering proteins from organisms ranging
from viruses to whales. DeepMind has designed this database in
partnership with the
European Molecular Biology Laboratory-European Bioinformatics
Institute
(EMBL-EBI).
AlphaFold2 also holds promise in drug discovery for neglected
diseases that affect very few people and, hence, do not attract
enough funding. Through its accurate structure prediction, the AI
model can help discover drugs for diseases such as Chagas disease
and Leishmaniasis, bypassing the need for expensive equipment and
laboratory work.
These are just a few of the many impactful applications of
AlphaFold2. It has dramatically advanced our understanding of
protein structures. It is a pioneering breakthrough achieved using
an interdisciplinary approach—combining the knowledge of
computation, machine learning, chemistry, physics, mathematics, and
biology. AlphaFold2 leads the way in which artificial intelligence
can help crack the mysteries of life.